News Story
The Buddy System: Human-Computer Teams
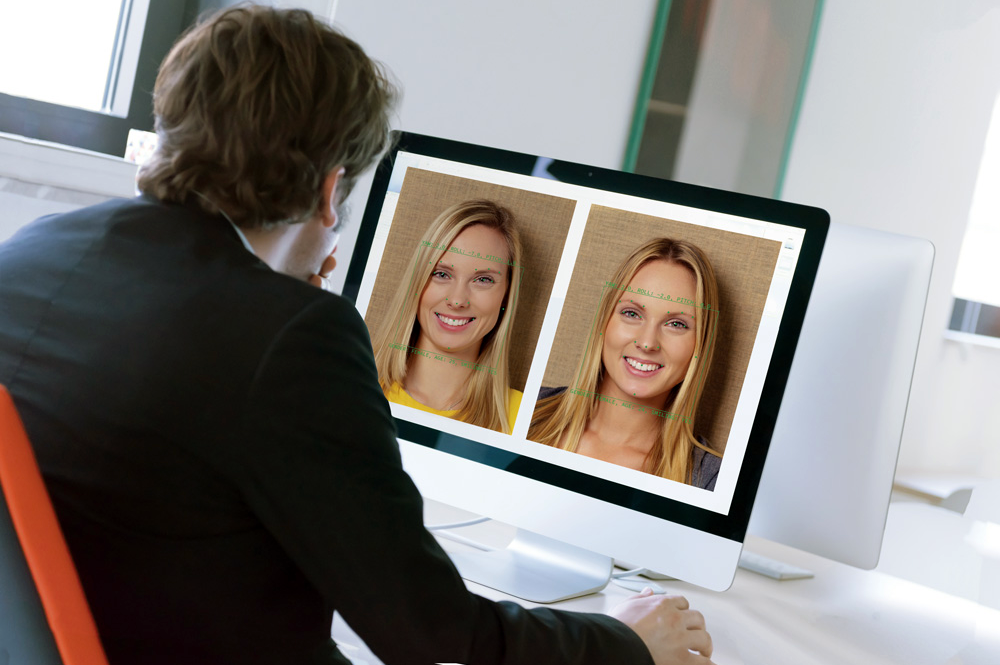
Is that him? Is she the one?
A prized attribute among law enforcement specialists, the expert ability to visually identify human faces can inform forensic investigations and help maintain safe border crossings, airports, and public spaces around the world.
The field of forensic facial recognition depends on highly refined traits such as visual acuity, cognitive discrimination, memory recall, and elimination of bias. Humans, as well as computers running machine learning (ML) algorithms, possess these abilities. And it is the combination of the two—a human facial recognition expert teamed with a computer running ML analyses of facial image data—that provides the most accurate facial identification, according to a recent 2018 study in which Rama Chellappa, Distinguished University Professor and Minta Martin Professor of Engineering, and his team collaborated with researchers at the National Institute of Standards and Technology and the University of Texas at Dallas.
Chellappa, who holds appointments in UMD’s Departments of Electrical and Computer Engineering and Computer Science and Institute for Advanced Computer Studies, is not surprised by the study results. “For this facial recognition task, like a lot of tasks in the future, humans will need a computer ML buddy to do it really well,” he says.
Pairing human expertise at decoding subtle cues such as emotional signals, context, and remembered experience with ML’s blazing computational power leverages the strengths of each. It also compensates for deficiencies. “Machines can mess up,” Chellappa says. “A dog can be mistaken for a traffic sign by a machine if the algorithm is given poor-quality data. Human judgment and experience can easily spot that kind of error and correct it.”
In the early days of computer vision research, Chellappa’s labs followed the common research approach of mathematically connecting inputs of data to obtain outputs that enabled them to explore the underlying physics of image formation and geometry of objects. Mapping the contours and appearances of a face, for example, was a common task.
All this changed in 2012. That’s the year the technique for layering input–output data emerged. This led to the ML approach known as deep learning, supported by an algorithmic configuration referred to as neural networks.
Deep learning affords greater ML accuracy than ever before by overcoming the constraints of limited amounts of data. The layering of data makes more data points available for analysis and powers more robust, accurate analyses of patterns and predictions. Around the same time, affordable advances in computing and graphics processing power also became available.
Combined, the confluence of the two—development of neural network deep learning and faster machine processing—led to the 2012 breakthrough that now powers technologies central to computer vision and facial recognition. And so much more, from driverless cars to voice recognition and chatbots.
“I call it the earthquake of 2012,” Chellappa says. “It completely turned our world upside down.”
Though deep learning contributes enormously to improved accuracy in facial recognition, Chellappa believes in the conclusion suggested by the 2018 study: the future is still human. Optimal accuracy in facial recognition and other ML-mediated tasks will likely come from humans and machines teaming up.
For example, he sees enormous potential to apply deep learning and the buddy system of human–computer teams to issues in medicine and human health. In a cardiac exam, the ML-enabled computer could make diagnostic suggestions from patterns in the patient’s heart data and help predict patients at risk for a cardiac event. The human medical professional would analyze the machine’s report and make the final diagnostic decision, and design the plan for how and when to treat.
Says Chellappa: “ML is remarkably powerful in identifying patterns and making predictions, especially when there is a lot of high-quality data, so I’m very excited about this deep learning potential in medicine to improve care. It’s an exciting time to be in computer vision and machine learning.”
Published March 27, 2019