News Story
Researcher’s Pacific Dive Spurred Innovations in Robotics with Machine Intelligence to Create Biodegradable Plastic Substitutes
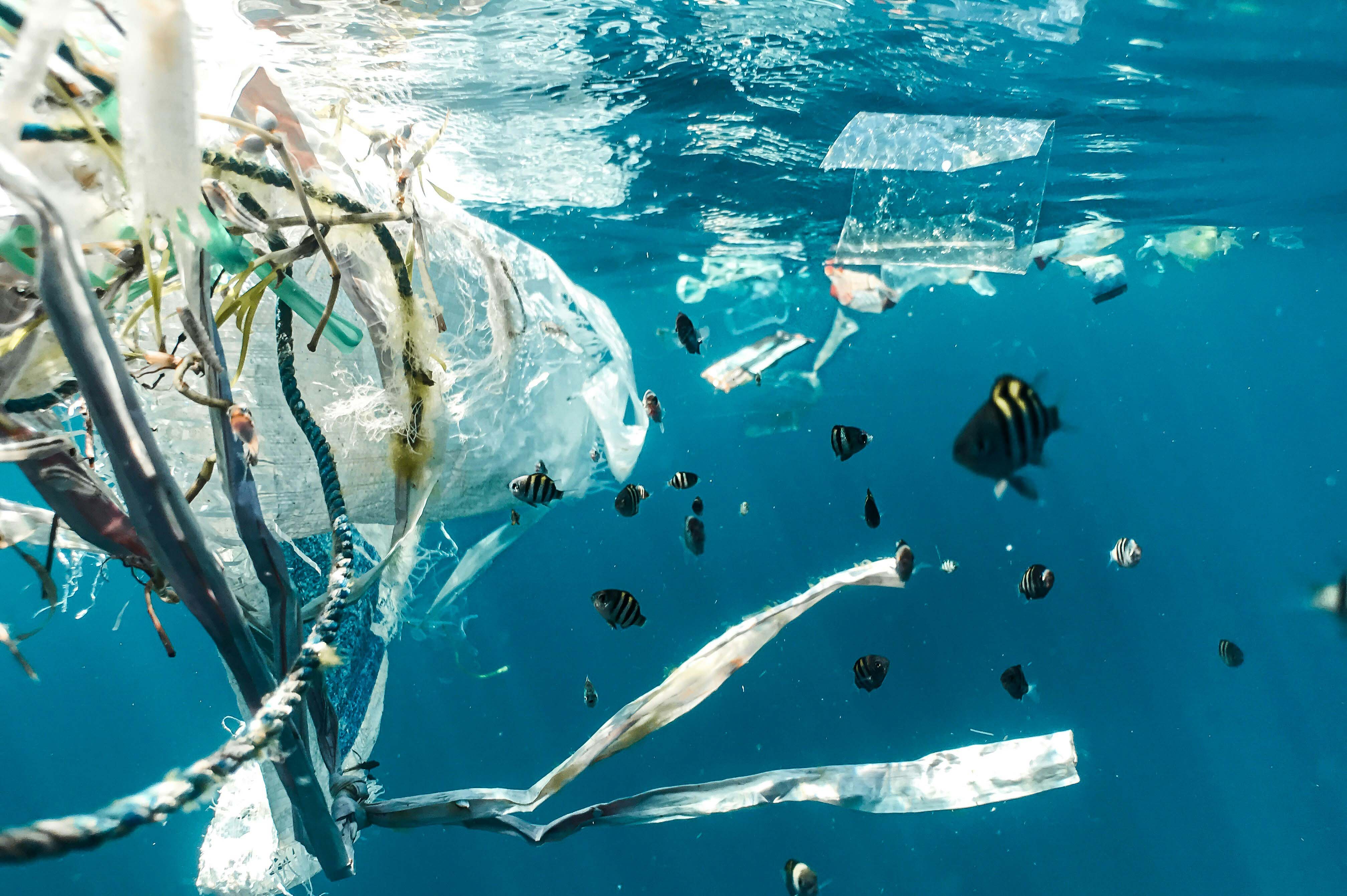
While exploring Western Pacific island Palau in 2019, Po-Yen Chen watched plastic films floating above the sea—attracting hungry fish in search of algae. Troubled by what he’d seen, the University of Maryland researcher thought he could use his expertise to find a solution.
“I started to think about how persistent plastics can be in natural environments, and when I established my research lab in Maryland, I immediately saw an application for my machine learning framework that I had been seeking for a while,” said Chen.
What resulted was a method to accelerate the discovery of new formulations for biodegradable plastic alternatives by leveraging artificial intelligence and robotics. Chen, an assistant professor in the Department of Chemical and Biomolecular Engineering (CHBE), described his approach to automate the design of natural substitutes for petroleum-based synthetic polymers in a study, published last Monday in Nature Nanotechnology.
Each year, about 300 million tons of plastics are produced globally, according to a United Nations estimate, and used throughout the economy. In addition to plastic pollution in water bodies resulting from poor waste management, the extraction, refinement and transportation of fossil fuels for plastic production generates over a billion tons of greenhouse gas emissions each year.
Chen sought out a way to identify substitutes that not only break down relatively quickly in the environment, but that replicate the attributes enabling the broad application of plastics, such as sufficient optical transparency, fire retardancy and strength. That meant he needed to be able to formulate and test a huge variety of samples with more speed and precision than any human could do it.
His technology works in two steps: First, an automated robot formulates hundreds of sample materials using four natural ingredients in varying ratios. These candidates are then examined for their ability to remain strong and freestanding—filtering out unqualified materials.
Then, in active learning cycles, researchers feed the machine learning model with sample data containing compositions and properties, and the model suggests a second set of sample materials to be synthesized. Following the cycles of formulation suggestion and experimental processes, this method allowed researchers to design biodegradable materials with programmed characteristics that could effectively replace plastics in wide uses.
They created eight transparent materials for a range of products, including name badge holders, file folders, shopping bags, lamp shades, inflatable pillows, non-flammable battery packaging and bags for chemical storage. Following the recent discovery, researchers will now focus on designing alternatives for food packaging.
Seeking to increase efforts to advance eco-friendly materials, Chen recently established MateriAI, a software firm for chemical and manufacturing companies seeking to adopt AI and machine learning tools to accelerate their assembly lines.
His work was supported by a UMD Grand Challenges Team Grant to advance climate change solutions along with mechanical engineering Professor Teng Li, who was a co-author of the study. The first author of this work is Tianle Chen, a CHBE graduate student.
Published March 25, 2024