News Story
Calculating Uncertainties in Chaotic Turbulent Flow Models
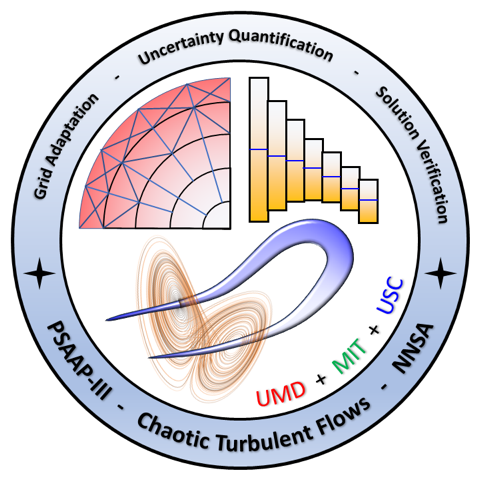
Computational science is a relatively new field. Historically, developing theories and devising experiments to test said theories underpinned the scientific process, but starting in the 1940s, with the invention of the computer, computational science—the ability to use computers to solve complex problems through equations and modeling—started becoming an accepted part of science and engineering practice.
“However, the challenge with current computational science paradigms is that while we have the ability to solve incredibly complicated equations, and make predictions for a variety of variables, what we generally don’t have is the ability to provide an error or uncertainty estimate on those predictions,” explained Johan Larsson, associate professor of mechanical engineering and expert in the field of fluid dynamics.
Fluid dynamics is one field that has leaned into the exploration of computational science, in particular, with applying it to the complex and chaotic world of turbulence. Turbulence touches almost every scientific field and facet of our daily lives. From weather predictions and patterns to vehicle fuel efficiency to airplane safety in take-off and landing, it is a powerful, and often unpredictable, force on the world around us.
Turbulence’s three-dimensional nature, and the fact that it requires a seemingly infinite number of variables to describe, means that creating models capable of accounting for all of those factors is difficult, costly, and fail to provide margins of error in their results.
“The response of nature is so complicated that it will not always do what you think, and small changes of variables in a chaotic system can have unpredictable or amplified affects,” explained Larsson. "That’s the chaos part, and you have to realize that there are always uncertainties."
Creating modeled equations that can estimate those uncertainties and generate margins of error in chaotic systems is exactly what Larsson and his colleagues Qiqi Wang (Massachusetts Institute of Technology) and Ivan Bermejo-Moreno (University of Southern California) aim to create.
To solve this seemingly impossible task, the team’s goal is to develop an adjoint equation for computational models that will not only enable simulations to account for the multitude of variables in a chaotic system, but also perform the task of a Monte Carlo type risk analysis to provide a margin of error, all in a single simulation.
The Monte Carlo simulation—the standard risk analysis method for calculating margins of error—relies on running thousands of simulations with slight changes in variables for each repetition; however, many current simulations are so complex in nature that the cost to compute those simulations enough times to obtain any statistically reliable margin of error would be prohibitively expensive.
Turbulence flow simulations account for almost half of the workload on some of country’s largest super computers, and according to Larsson, a single simulation can use ten percent of the computer’s capacity over the span of several weeks to solve for a single flow condition.
“Most engineering problems of real interest, they have hundreds or more parameters specifying the flow condition that we don’t know the exact value of, so that’s why our method, if it works, could be game changing," said Larsson. “Our research aims to not only develop ways to obtain an approximate uncertainty estimate, but to do so at a much lower cost.”
Larsson laid the groundwork for this research through preliminary work and feasibility checks using the University of Maryland’s DeepThought2, a high-performance computing (HPC) cluster housed on campus. While the state of Maryland is home to several large supercomputers, including the BlueCrab HPC cluster housed at the Maryland Advanced Research Computing Center (MARCC), UMD researchers only have access to about 15% of that resource. This means researchers like Larsson frequently have to rely on out-of-state resources to complete their work, like the Theta supercomputer at Argonne National Laboratory in Chicago.
When asked why no one has tried to solve this particular problem before, computational capacity aside, Larsson replied, “Because it’s really, really difficult. The beauty of computational science is that it is so inherently multi-disciplinary. Everyone talks about that word, but this is multi-disciplinary in that you have to be really comfortable and good at your engineering discipline, like turbulence, and in applied math, and a little bit in computer science. It’s unusual for people to have expertise in all three.”
If Larsson and his colleagues are able to leverage this unique confluence of expertise and succeed, the resulting methodology could be used in a very wide range of scientific and engineering disciplines, by enabling simulation-based critical decision-making.
The team’s research is funded through a five-year, 2.5 million dollar grant awarded from the U.S. Department of Energy’s Advanced Simulation and Computing (ASC) Predictive Science Academic Alliance Program (PSAAP)—part of the National Nuclear Security Administration (NNSA)—that focuses on the emerging field of predictive science.
The grant not only provides the research funding, but the team will have access to some of the country’s fastest super computers housed in Los Alamos National Laboratory, Lawrence Livermore National Laboratory, and Sandia National Laboratory. In addition, they will work with a technical steering committee comprised of scientists currently working in the area of turbulence simulations.
“It’s not only an opportunity for them to learn from us, but it also gives us a chance to learn from them and to understand what they care about,” adds Larsson. “The NNSA really wants to make sure that what we are able to accomplish crosses over and is put into practice.”
Learn more by visiting the team’s website, Turbulenza.
Published October 6, 2020